
MADNESS: Artificial intelligence-assisted methodology for the discovery of new materials in molten salts
Coordinator: David PORTEHAULT
LCMCP – Laboratoire de Chimie de la Matière Condensée de Paris
(UMR 7574 Sorbonne Univ./CNRS/Collège de France/Univ. Paris sciences et lettres)
Keywords: New materials, Liquid-phase synthesis, Molten salts, Intermetallics and compounds, X-ray diffraction in situ, Crystal structure prediction, Supervised machine learning, Graph neural networks, Electrocatalysis
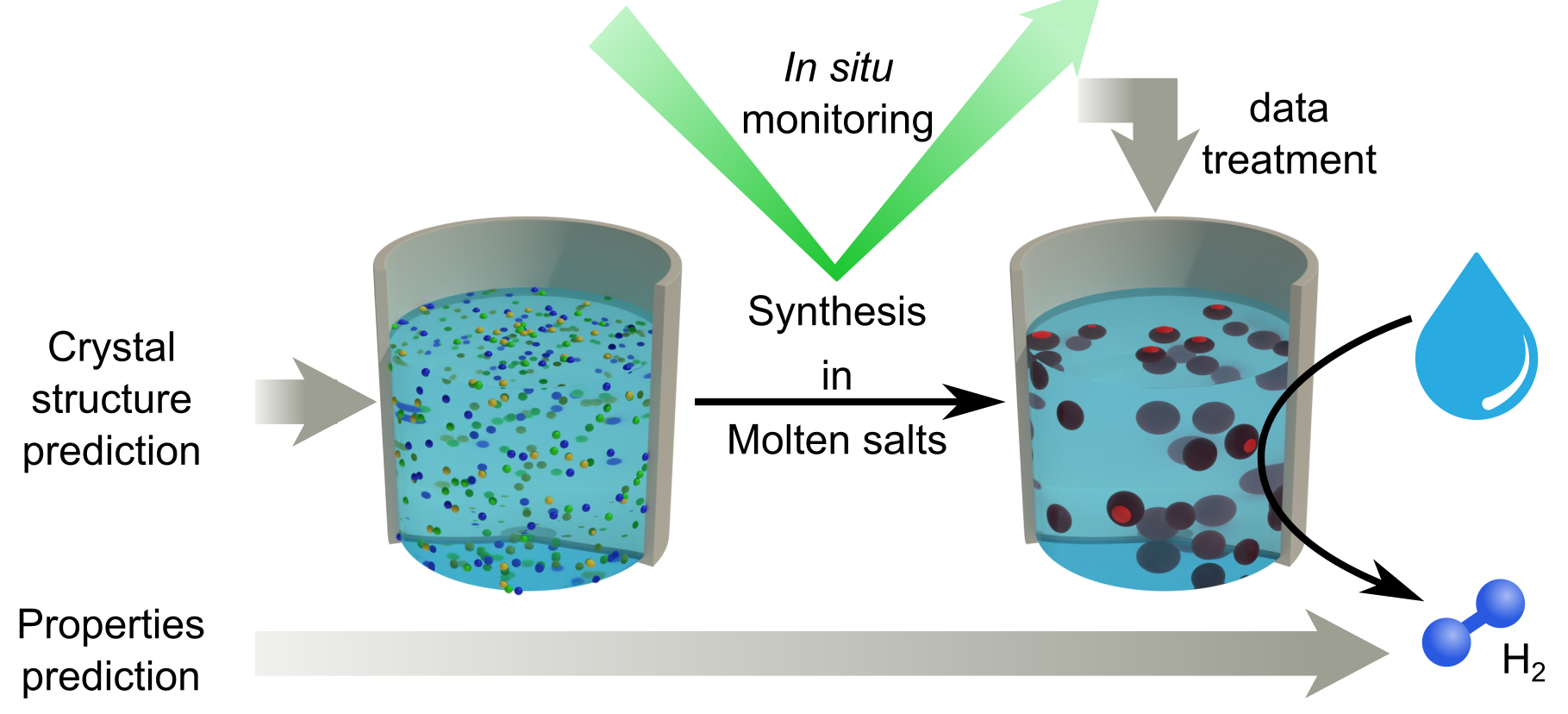
The aim of the MADNESS project is to combine artificial intelligence and inorganic synthesis to answer a simple but highly complex question: how can we accelerate the search for materials using synthesis techniques that are difficult to automate?
We will focus on molten-salts synthesis as a case study, an original method whose capacity to open the way to new materials has been emerging for several years, but which is not suited to automation and high-throughput screening. More specifically, we will search for materials capable of replacing precious metals in the electrocatalysis of dihydrogen production from water, by focusing on promising compounds of transition metals and noncritical elements whose chemical space is mostly a virgin field, because they are very difficult to synthesize. We will develop a synthesis methodology relying on two pillars:
The first pillar consists in taking the most from each synthesis, by considering each experiment as a large data source to screen some of the most central experimental parameters. To do so, we will use X-ray diffraction under synchrotron radiation with the aim of monitoring in situ the syntheses, and we will develop machine learning (ML) for automatic analysis.
The second pillar is the prediction of new compounds and of phase diagrams, for unexplored or poorly understood chemical systems by using generative techniques to accelerate the search of new compounds and anticipate the electrocatalytic properties for H2 production from water, which will be measured a posteriori.
The MADNESS project will draw on DIADEM‘s infrastructure to provide a methodology for accelerating materials discovery via molten-salts synthesis and, more generally, via other synthesis methods unsuitable for automation. Its originality lies in the methods we want to develop for addressing its objectives, where AI will assist experimental work to screen, predict, anticipate and realize new functional materials. MADNESS will enable, for the first time, an intimate link between inorganic synthesis (LCMCP), high-end characterization (SOLEIL), AI-assisted data treatment (IMN) and AI-assisted crystal structure prediction (LINK), so thatpredicted materials can be realized experimentally. By delivering a methodology for AI-assisted synthesis, the MADNESS project will help to democratize artificial intelligence approaches among the broad community of inorganic materials synthesis chemists.