
MOFlearning: Accelerating the design of MOFs through a Machine Learning assisted High-throughput methodology
Coordinator: Christian SERRE
IMAP – Institut des MAtériaux poreux de Paris
(UMR 8004 ENS Paris/CNRS/Univ. Paris Sciences Lettres/ESPCI Paris)
Keywords: MOFs, machine learning, high-throughput
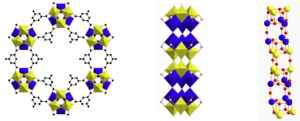
Crystalline porous hybrid solids such as Metal Organic Frameworks (MOFs) are of interest for a wide range of applications (separation, catalysis, detection, biomedicine, etc.). However, their synthesis is most often not controlled, which makes it difficult to produce them “custom” according to the targeted property.
The state of the art relies on ‘big data’ approaches with few guarantees that the selected materials can be synthesized. This has led to some success in large-scale prediction of structure-activity correlations but for a very limited number of properties (e.g. in adsorption). In addition, high-speed modeling of other physical or chemical properties (at the Classical or quantum level) is not yet an established methodology and would require other developments to support the expansion of databases as learning tools for artificial intelligence type approaches. This demonstration project aims to establish for the first time, in the field of MOFs, a methodological approach guided by high-throughput synthesis and characterization methods.
We will rely on a series of existing MOFs with adequate structural and chemical diversity and the systematic evaluation of their adsorption/separation properties of model gas molecules (CO2, CH4, N2, noble gases, etc.), mainly polycarboxylates or metal phosphonates with a high degree of oxidation, functionalized by polar or apolar groups, which can be easily produced and activated on the scale of a few grams. This experimental effort will be complemented by high-throughput prediction of their chemical and physical properties to create enriched databases, with indicators as learning means for statistical algorithms to identify key descriptors, coupled with generative algorithms to explore the space of new phases and guide future discoveries. Secondly, based on a new high-throughput synthesis and characterization platform and new predictive models, for 1 or 2 applications of interest, we will identify and synthesize new high-performance MOFs.